NCT Data Science Seminar
Das NCT Data Science Seminar ist eine campusweite Initiative, die führende Forscher im Bereich der Datenwissenschaft zusammenbringt, um methodische Fortschritte und medizinische Anwendungen zu diskutieren.
Um stets über kommende Vorträge informiert zu bleiben, abonnieren Sie unseren mailing list.
Upcoming & Recent Talks
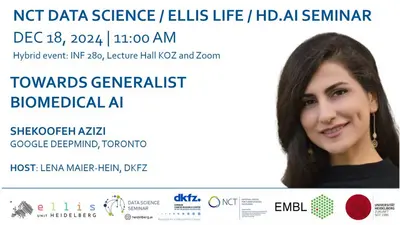
Foundation models have changed how we develop medical AI. These powerful models, trained on massive datasets using self-supervised learning, are adaptable to diverse medical tasks with minimal additional data and paved the way for the development of generalist medical AI systems. In this talk we will explore the capabilities of these models from medical image analysis, to polygenic risk scoring, and aiding in therapeutic development. Additionally, we will discuss the future of generalist and generative models in healthcare and science.
Bio:
Shekoofeh (Shek) Azizi is a staff research scientist and research lead at Google DeepMind, where she focuses on translating AI solutions into tangible clinical impact. She is particularly interested in designing foundation models and agents for biomedical applications and has led major efforts in this area. Shek is one of the research leads driving the ambitious development of Google's flagship medical AI models, including REMEDIES, Med-PaLM, Med-PaLM 2, Med-PaLM M, and Med-Gemini. Her work has been featured in various media outlets and recognized with multiple awards, including the Governor General's Academic Gold Medal for her contributions to improving diagnostic ultrasound.
Recorded Talks
Hosts
3 Mitarbeiter:innen
-
Prof. Dr. Lena Maier-Hein
-
Prof. Dr. Klaus Maier-Hein
-
Prof. Dr. Oliver Stegle
Kontaktieren Sie uns
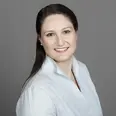