Knowledge Modeling

Dr. Wahyu Wijaya Hadiwikarta
Head of Knowledge Modelling & RadPlanBio
In the Knowledge Modeling working group, we develop methodologies for extracting knowledge from integrated, linked preclinical and clinical radiotherapy trial datasets. These datasets, collected under standardized protocols, exhibit inherent heterogeneity due to structural complexity and multimodal characteristics. To address this, we design models that capture stochastic variability within a semantically linked knowledge graph, enabling robust and interpretable data integration.
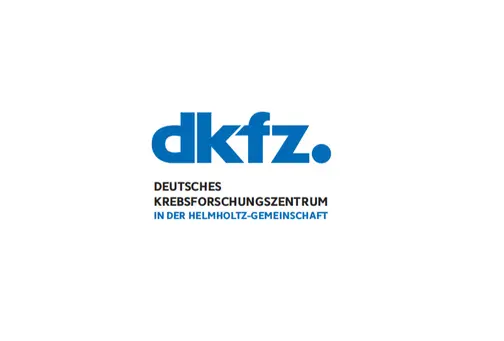
Our Research
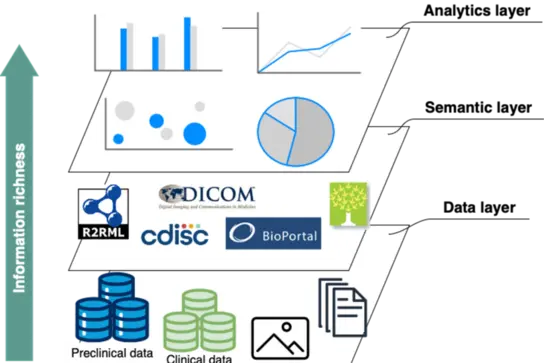
We apply semantic methodologies to integrate preclinical and clinical datasets into knowledge graphs (KG), creating a structured, interoperable representation of biomedical information. By leveraging domain-specific ontologies, we enhance data standardisation and ensure compliance with FAIR principles (Findable, Accessible, Interoperable, Reusable), facilitating knowledge discovery and supporting translational research. Our approach is applied to datasets from multiple studies hosted on the German Cancer Consortium’s (DKTK) RadPlanBio platform, where we systematically organise and link data across preclinical and clinical domains. Preclinical datasets adhere to the SEND standard for nonclinical data tabulation and are enriched using the PTRO ontology, which is currently under active development. Expanding these FAIRification efforts to clinical data will further enhance data integration, accessibility, and usability, driving advancements in personalised medicine and fostering innovation in therapeutic development.
To extract deeper insights, we develop an analytical framework based on the concept of propagators from quantum physics, trying to leverage the structured representation of knowledge to infer cancer progression mechanisms. The complete set of numerical and non-numerical data corresponding to a single patient at a given point in time provides a snapshot of the patient’s state, representing an abstract "position" within the space of all possible conditions. As time progresses, this state undergoes observable changes, which we hypothesise can be described by a transfer function, called propagator. This approach enables a systematic modelling of disease dynamics, offering a principled way to predict disease trajectories and identify critical transitions in cancer progression.

Given the observed data of what we know about the subject’s state at given times and a set of rules (Γ) for evolution, the propagator (G) considers all possible paths the subject could have taken that are compatible with our limited knowledge.
By carefully and deliberately adjusting the aforementioned set of rules underlying the propagator and estimating the likelihood given our data, we try to infer the most likely rules of cancer growth. With these rules at hand, we want to understand and predict cancer growth and survival effectively, ultimately aiming to help with treatment strategy decisions.
Get in touch with us
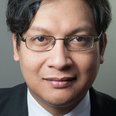
Dr. Wahyu Wijaya Hadiwikarta
Head of Knowledge Modelling & RadPlanBio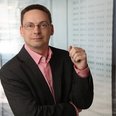