Digital Oncology
Digital Oncology at DKFZ pioneers cutting-edge data science, driving breakthroughs by integrating flagship data infrastructures with methodological advances in bioinformatics, omics, imaging, clinical data, as well as multimodal, explainable, and generative AI. Our mission: tackle big questions in oncology by uniting the computational expertise across the center.
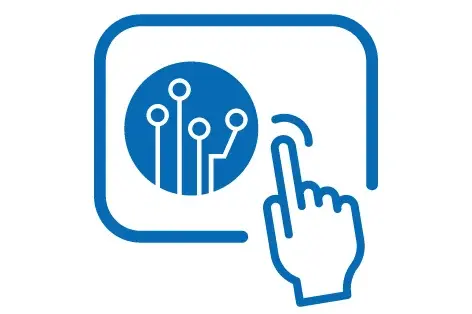
Digital Oncology at DKFZ integrates cutting-edge data science with cancer research. With a team of approximately 250 researchers across nine divisions, the cross-topic aims to create benchmarks for bioinformatics and imaging while providing and managing important research (data) infrastructures. This comprehensive “end-to-end” strategy bridges core teams as well as external partners, supporting computational scientists in experimental and clinical settings through specialized training and exchange platforms.
With cross-topic exchange as a guiding principle, Digital Oncology collaboration serves as the thread that binds diverse disciplines, creating a rich tapestry of innovation in cancer research. The analytical expertise of data scientists is needed to intertwine with the expertise of health and life scientists, where each exchange represents a new link in a united effort to understand, prevent, and treat cancer.
Coordinators
-
Prof. Dr. Martin Lablans
-
Prof. Dr. Klaus Maier-Hein
-
Prof. Dr. Lena Maier-Hein
-
Prof. Dr. Oliver Stegle
Faculty
-
Priv. Doz. Dr. Titus Brinker
Digital Prevention, Diagnostics & Therapy Guidance
-
Prof. Dr. Benedikt Brors
Applied Bioinformatics
-
Prof. Dr. Moritz Gerstung
AI in Oncology
-
Prof. Dr. Angela Teresa Filimon Goncalves
Molecular & Computational Prevention
-
Prof. Dr. Thomas Höfer
Theoretical Systems Biology
-
Prof. Dr. Annette Kopp-Schneider
Head
-
Prof. Dr. Jan Korbel
Mechanisms of Genomic Variation & Data Science
-
Priv. Doz. Dr. Karl-Friedrich Kowalewski
Intelligent Systems and Robotics in Urology
-
Prof. Dr. Martin Lablans
Federated Information Systems
-
Prof. Dr. Klaus Maier-Hein
Medical Image Computing
-
Prof. Dr. Lena Maier-Hein
Intelligent Medical Systems
-
Prof. Dr. Stefanie Speidel
Translational Surgical Oncology
-
Prof. Dr. Oliver Stegle
Computational Genomics & System Genetics
PI | Division | Research Topic | ||
---|---|---|---|---|
Moritz Gerstung | AI in Oncology | FS-B | core AI | omics |
Benedikt Brors | Applied Bioinformatics | FS-B | bioinformatics | omics |
Annette Kopp-Schneider | Biostatistics | FS-C | statistics | |
Oliver Stegle | Computational Genomics & System Genetics | FS-B | core AI | omics |
Titus Brinker | Digital Prevention, Diagnostics & Therapy Guidance | FS-C | core AI | imaging |
Martin Lablans | Federated Information Systems | FS-E | platforms | |
Lena Maier-Hein | Intelligent Medical Systems | FS-E | core AI | imaging |
Karl-Friedrich Kowalewski | Intelligent Systems and Robotics in Urology | FS-E | surgery | |
Klaus Maier-Hein | Medical Image Computing | FS-E | core AI | imaging |
Angela Goncalves | Molecular & Computational Prevention | FS-C | bioinformatics | omics |
Thomas Höfer | Theoretical Systems Biology | FS-B | core AI | omics |
Stefanie Speidel | Translational Surgical Oncology | FS-E | surgery |
Research Divisions
The Gerstung lab develops and uses AI to study cancers. Our data-driven approach helps reach a deeper understanding of cancer biology, identify cancer risks and improve diagnostic workflows. Termed the emperor of maladies, the mechanisms causing cancer range from genomic alterations, cell and tissue biology to behavioural and environmental exposures. A comprehensive assessment is thus warranted to recognise each tumour's unique characteristics. While rich molecular 'omics' technologies chart many of these layers, the amounts of data derived from such assays require bespoke algorithms to derive mechanistic and predictive insights. Our approach thus utilises multimodal AI to integrate data from health records, imaging and omics assays.
Gerstung Working GroupWe are developing and applying bioinformatical algorithms to data from cancer genomics and epigenomics. Our aims are to elucidate causes of cancer and cancer progression, understand evolution of resistance against therapies, and develop AI-based diagnostics for rational choice of cancer therapies. By analyzing data from single-cell sequencing assays, we aim to model and understand the interactions between tumor cells and the immune system. We are part of international networks like the International Cancer Genome Consortium, the Pan-cancer Analysis of Whole Genomes Project, the Pan-prostate Cancer Group, and the International Human Epigenome Consortium. We also contribute to national infrastructures, e.g. the German Network on Bioinformatics Infrastructure (de.NBI), and the German Human Genome-Phenome Archive (GHGA). We are closely connected to clinical trials in precision oncology, and are part of the German Cancer Consortium (DKTK).
Brors Working GroupThe mission of the Division of Biostatistics is to support DKFZ scientists in performing and publishing excellent reproducible research. Adequate experimental design and analysis strategies are rarely available ‘off the shelf’ but must be developed and tailored to the specific problem in collaboration with the biomedical researcher. Our methodological research activities cover a wide range of biostatistical topics, often motivated and interlinked with long-standing collaborations within and outside the DKFZ, including a large number of clinical trials. Major areas of current research interest include: design and analysis of clinical trials, both in the frequentist setting as well as in the Bayesian framework; identification of prognostic and particularly predictive factors from clinical and molecular data; optimal design and analysis for dose-response relationships, with a focus on combination of substances; measuring dependence between sets of random variables for various data types.
Kopp-Schneider Working GroupMolecular variation has long been linked to phenotypic changes, including human diseases, yet dissecting the underlying mechanisms remains challenging. Growing sample sizes and technological advances demand novel analytical strategies and tools that scale to datasets with millions of observations and account for spatial and temporal dependencies. Our laboratory develops and applies computational approaches to study molecular variations and their phenotypic consequences. We aim to understand how our genetic backgrounds shape phenotypic traits or cause disease, how genetic and environmental factors integrate at different molecular layers, and how molecular states vary between individual cells. To address these questions, we use statistical inference and machine learning as core tools. Examples of our work include efficient parameter inference in models to probe genetic associations and methods for dimensionality reduction.
Stegle Working GroupThe main goal of the group is the development of robust and interpretable digital biomarkers to improve prevention, non-invasive early detection, diagnostic, and therapeutic approaches. A 20-member, almost fully externally funded team from the fields of medicine, molecular biology and informatics/data science focuses on identifying relevant patterns in patient data and increasing the explainability and robustness of deep learning-based classifications. We see software systems as part of clinical teams for more efficient patient care and at the same time as a tool for effective prevention and early detection.
Brinker Working GroupWe build bridges among institutions with GDPR-compliant federated data management solutions, including the “Mainzelliste” for pseudonymization and record linkage, several federated search solutions and the “Bridgehead” for controlled data sharing. These form the backbone of the German Cancer Consortium (DKTK), lung cancer patients data collection in the National Network Genomic Medicine (nNGM) and the “Sample Locator”, which allows federated search across 16 European biobanks (BBMRI-ERIC). As a bridge division to the University Medicine Mannheim, we foster data-driven collaborations as part of the DKFZ Hector Cancer Institute’s novel approach to data sharing with the Medical Informatics Initiative.
Lablans Working GroupOur group has three areas of expertise. One focus is on precision oncology, with bioinformatic analyses and identification of targetable lesion in real patient data for molecular tumor boards. In particular, we are the bioinformatic backbone for the programs MASTER (Molecularly Aided Stratification for Tumor Eradication Research) and CATCH (advanced-stage / metastatic breast cancer) / COGNITION (early breast cancer) at NCT Heidelberg. A second focus is translational research with multi-omics cohort analyses and pattern recognition, as well as molecularly stratified clinical trials. A third focus is the development of algorithms including AI and ML, bioinformatics tools, pipelines and methods for analysis of next generation sequencing data, multi-omics integration, and single cell data analysis. Together with partners, we are strongly contributing to the emerging field of cellular interactions by developing data analysis methods for spectral flow cytometry and imaging flow cytometry.
Computational Oncology GroupArtificial intelligence (AI) is set to revolutionize various areas of everyday life. In healthcare, however, its integration into routine procedures and impact on patients are still limited. The mission of the Division of Intelligent Medical Systems is to tackle the unique challenges of medical imaging AI to generate lasting patient benefit. A particular focus of our research lies in the surgical application domain. Currently, patient outcome is heavily influenced by the surgical team's experience, with many potential complications being avoidable. To address such major socioeconomic problems and consistently elevate patient outcome beyond current standards, our multidisciplinary teaam works on intelligent medical systems in close collaboration with clinicians. Key methodological challenges are related to the generalization of AI methods across devices, patient populations and hospitals, high data variability in an inherently sparse data regime, multimodal data integration, incorporation of prior knowledge into models, and the meaningful validation of AI systems under real-world clinical conditions.
Lena Maier-Hein Working GroupThe Division of Medical Image Computing (MIC) leads research in machine learning and information processing to enhance cancer patient care through systematic image data analytics. We integrate imaging information from technologies like MRI and CT with clinical and biological data. As co-coordinator of the Helmholtz Imaging Platform, we drive computer science innovations, particularly in semantic segmentation, object detection, unsupervised learning, and probabilistic modeling. Our advanced research software supports scalable data analysis in federated settings, forming the backbone of clinical research networks such as NCT, DKTK, and CCE. Collaborating with clinical partners, we aim to translate AI advancements into practical clinical applications. We are committed to creating robust and generalizable algorithms that endure the test of time, maintaining their relevance in an ever-changing technological landscape. Committed to open science, we maintain several open-source projects, sharing our progress with the community to foster collaboration and synergies.
Klaus Maier-Hein Working GroupOur group aims to understand how mutant clones arise and expand in epithelial tissues during aging and immediately preceding the development of malignancy, with a long-term view of improving the prevention and early detection of cancer. We are particularly interested in using tissue-level approaches to investigate the relative contribution of mutations and microenvironment-driven-promotion to carcinogenesis. To do so we design large-scale experimental genomic experiments which we analyse with ML/AI and statistical modelling approaches.
Goncalves Working GroupWe investigate the initiation of malignant transformation in the blood, and other developing or renewing tissues. To this end, we develop quantitative and predictive models of stem cell dynamics and somatic evolution, and of how the two processes interact. These computational models are based on data from human tissue samples, or from genetic mouse models for cell barcoding, lineage tracing and somatic evolution. We develop long-term collaborations to tightly link modeling and computation with experiment. Besides stem cell dynamics and somatic evolution, a specific focus of the group is on the clonal dynamics of adaptive immune responses. Among other insights, we have shown that T cell memory develops early during an immune response, identified multipotent progenitors as the active stem cells of steady-state and emergency hematopoiesis, and found that genetic evolution of many tumors starts years or even decades before diagnosis.
Höfer Working GroupInfrastructure Leads
-
Dr. Claudia Galuschka
ITCF
-
Dr. Ivo Buchhalter
ODCF & de.NBI
-
Prof. Dr. Elisa May
Enabling technology - Core Facilities & NFDI4Bioimage
-
Dr. Christian Busse
NFDI4Immuno
-
Dr. Jan Eufinger
GHGA
-
Alexander Knurr
CTO-SUDO
-
Dr. Daniel Kraft
NAKO Central Data Management
-
Dr. Marco Nolden
HMC Hub Health
Infrastructures & Service Units
The IT Core Facility (ITCF) at DKFZ supports all staff by ensuring optimal IT usage, crucial for research and administration. IT is vital due to large-scale data from genome analysis, radiological imaging, and increasing digitalization of administrative tasks. IT security is essential, as personal data processing is critical for national and international research collaborations. ITCF provides central services, support, and customized solutions.
Key working groups include:
- Network: Manages data networks, internet connections, and security services.
- Server and Compute: Operates scientific computing platforms like the DKFZ Cloud and in close collaboration with ODCF GPU clusters. Central servers, mail and file service, printing services, and data storage are also tasks of the working group.
- Software Systems: Handles user management, database services, and resource management.
- Application Development: Builds web applications and APIs for data exchange.
- Desktop Services: Supports users with devices, desktop environments, and printing.
- Partner Sites: Aids DKFZ partner networks with IT connections and coordination.
- IT Security: Oversees operational monitoring and responses to security incidents
IT plays a pivotal role in advancing cancer research and life sciences.
ITCFThe Omics IT and Data Management Core Facility (ODCF) at the DKFZ provides a comprehensive platform for high-throughput computing, data management, and basic bioinformatics services. Through the One Touch Pipeline (OTP), researchers can easily set up projects, submit sequencing and other research data, and manage metadata. Secure file storage, user access controls, and project sharing capabilities streamline collaboration. The ODCF supports a variety of workflows, from single-cell data processing to alignment, quality control, and variant calling pipelines. Data submission tools, including the ODCF Guide, ensure consistent and validated metadata, while integration with external repositories like GHGA facilitates broader data sharing. The DKFZ compute cluster (operated in close collaboration with the DKFZ IT Core Facility) delivers scalable computing resources under IBM LSF, supported by user documentation, storage options, and specialized services like IGV-Linker for genome visualization. Additionally, RStudio Workbench and JupyterHub offer flexible environments for interactive data analysis, empowering researchers with end-to-end bioinformatics solutions.
ODCFThe de.NBI Cloud at DKFZ, operated by the Omics IT and Data Management Core Facility (ODCF), provides a high-performance, scalable environment tailored for life science data analysis. As part of the German Network for Bioinformatics Infrastructure (de.NBI), it offers Infrastructure-as-a-Service capabilities that enable researchers to deploy virtual machines, manage large datasets, and conduct complex bioinformatics workflows in a secure setting. The platform leverages OpenStack technology or a Kubermatic based managed Kubernetes, ensuring a flexible, user-friendly experience for academic and non-profit partners. Through extensive CPU and GPU resources, the DKFZ de.NBI Cloud supports computationally intensive tasks like genomic data processing, single-cell analysis, and machine learning. By fostering collaborative, cross-institutional access to robust computing infrastructure, the de.NBI Cloud at DKFZ drives innovation in biomedical research, ultimately improving our understanding of diseases and advancing therapeutic discoveries.
de.NBI CloudThe Enabling Technology Department integrates several DKFZ core facilities producing large -omics data sets for cancer research. Among them are Next Generation Sequencing, Proteomics, Single Cell OpenLab, Flow Cytometry and Light Microscopy. Additionally, the Department plays a role in advancing biomage data management through its involvement in the DFG project "Information Infrastructure for BioImage Data Management – I3D:bio", and the consortium "NFDI4BioImage" within the National Research Data Infrastructure NFDI.
Enabling TechnologyThe immune system plays a fundamental role in health and disease and efficiently protects vertebrate hosts from infections and cancer. However, failures in its regulation can cause autoimmunity, allergy, immunodeficiencies and malignancies. To understand the mechanisms underlying these processes and how they can be manipulated for the benefit of humans and animals, immunologists use a wide range of experimental methods. The efficient handling of thereby generated data, so that they meet the scientific demands for reproducibility and reusability, is currently one of the key challenges for immunological research.
As one of the 26 NFDI (https://www.nfdi.de) consortia, NFDI4Immuno aims to initiate and shape the necessary transformation process together with its community. DKFZ and its 14 partner institutions (nine co-applicants and five participants) ensure the broad anchoring in the scientific community as a whole as well as in the respective thematic domains.
GHGA – The German Human Genome-Phenome Archive – is building a secure national omics data infrastructure, enabling the use of human genome data for research purposes while preventing data misuse. GHGA is funded via the German program for research data infrastructures (NFDI) and is coordinated at the DKFZ.
It serves as German's national node both within the federated European Genome-Phenome Archive (EGA) as well as the German node in the European Genomic Data Infrastructure (GDI). Nationally it has been mandated as the data infrastructure for secondary use of genomics and related data but key projects such as the German National Cohort (NAKO) and the German model project genome sequencing (genom.de).
The Secondary Use of Data in Oncology (SUDO) working group within the Clinical Trial Office supports DKFZ scientists and collaboration partners in utilizing clinical trial data beyond the original research questions. The group develops and operates innovative software solutions while ensuring compliance with regulatory and data protection requirements and adhering to the FAIR Data Principles, which promote the sustainable use of research data.
An additional key project of the working group is the development of the Knowledge Connector—a software solution designed to support molecular tumor boards. This initiative is carried out in close collaboration with the DKFZ’s Translational Medical Oncology (TMO) department.
The NAKO central data management aims to ensure efficient and standardized data collection, the guarantee of data integrity and quality and the best possible implementation of data protection and data security as well as the ethics concept; it ensures the central compilation and integration of all locally collected data. A data integration center with two sites (DKFZ Heidelberg and University of Greifswald) is responsible for the development and operation of standardized electronic data collection tools, efficient integration and long-term storage of data from all study centers. A transfer unit handles the trans-fer of data to scientific users.
The Helmholtz Metadata Collaboration (HMC) aims to improve the quality and usability of research data through standardized metadata. HMC is part of the Helmholtz Information and Data Science framework. It develops and implements novel concepts and technologies for sustainable research data handling through high-quality metadata. Its main goal is to make the depth and breadth of research data produced by Helmholtz Centres findable, accessible, interoperable, and reusable (FAIR) for the whole science community.
The HMC Hub Health is anchored at DKFZ and connects local activities in Digital Oncology with other Helmholtz centers in the research field of health. By linking with national and international networks like NFDI and EOSC, the HMC Hub Health facilitates the exchange of knowledge, expertise, and best practices, contributing to the advancement of the Digital Oncology program.
Seminar Series
Initiatives & Services
Get in touch with us
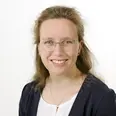
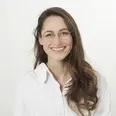