Computational Genomics and Systems Genetics
- Functional and Structural Genomics

Prof. Dr. Oliver Stegle
Division Head
Our research group develops and applies computational approaches to study molecular variations and their phenotypic consequences, such as the development of cancer.
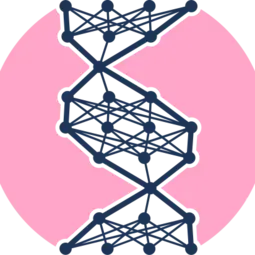
Our Research
How does our genetic background shape phenotypic traits or cause cancer? How are genetic and environmental factors integrated at different molecular layers, and how variable are molecular states between individual cells?
We use statistical inference and machine learning as our main tools to address these scientific questions. Growing sample sizes and technological advances demand novel analytical strategies and tools that scale to datasets with millions of observations and account for spatial and temporal dependencies. We develop foundational methods in statistics and machine learning, including efficient parameter inference in models to probe genetic associations and methods for dimensionality reduction.
Research Themes
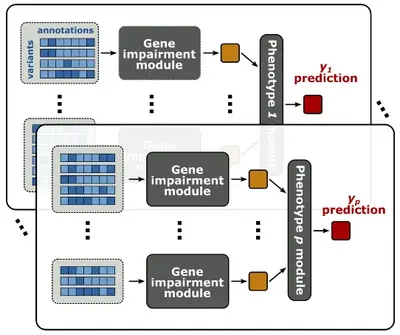
We develop statistical methods to dissect often overlooked dimensions of the genotype-phenotype relationship, including genetic effects of rare variants and indirect genetic effects.
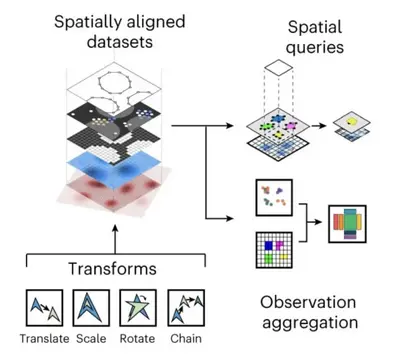
We develop foundational methods to integrate high-dimensional molecular profiles assayed in bulk or at the single-cell level. This includes innovations to allow for integrating multi-omics readouts across time and space, as well as methods for spatial omics technologies.
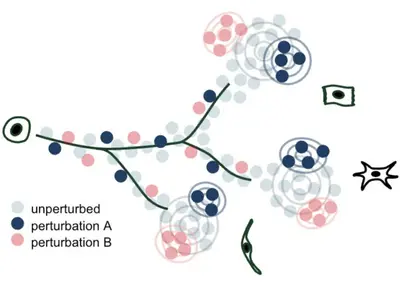
We develop methods to model genetic perturbation effects and infer causality. We leverage opportunities provided by engineered perturbations using CRISPR screens combined with single-cell readouts.
Team
We are an interdisciplinary and international team jointly hosted at the German Cancer Research Center (DKFZ) and the European Molecular Biology Laboratory (EMBL) in Heidelberg. In our team we promote openness and collaboration, and value kindness in everything we do. We take pride in every single member and their achievements and are eager to foster their scientific development.
-
Prof. Dr. Oliver Stegle
Division Head
-
Rama Abdulhamid
-
Alexander Aivazidis
-
Thibault Bechtler
-
Eva Sabine Blum
-
Dr. Brian Clarke
-
Daniel Dimitrov
-
Kevin Domanegg
-
Fritz Donald Hansen
-
John Andrew Hawkins
-
Elyas Heidari
PhD Student
-
Dr. Florian Heyl
-
Jan Gunnar Gleixner
Doktorand
-
Dr. Ilia Kats
-
Sameesh Kher
-
Dr. Santiago Lago
-
Jiawei Luo
-
Dr. Jusung Lee
-
Luca Marconato
-
Katharina Mikulik
-
Abdul Moeed
-
Marcel Mück
-
Dr. Hakime Öztürk
-
Martin Rohbeck
-
Manu Saraswat
-
Felix Schneider
-
Stefan Schrod
-
Ezgi Sen
-
Danai Vagiaki
-
Florin Walter
Open Positions
We are always looking for new talent to strengthen our team!
To apply for a postdoctoral position or PhD position in our group, please refer to the DKFZ Postdoctoral Fellowship and the DKFZ International PhD Programme.
If you do not apply through one of the specific openings mentioned above, feel free to send your CV, a letter of motivation and ideally 2 references in a single pdf directly Oliver Stegle.
We are also pleased to offer opportunities for your BSc or MSc thesis or an internship in our lab. If you are interested, please contact us with a CV and a motivation letter tailored to our lab interests.
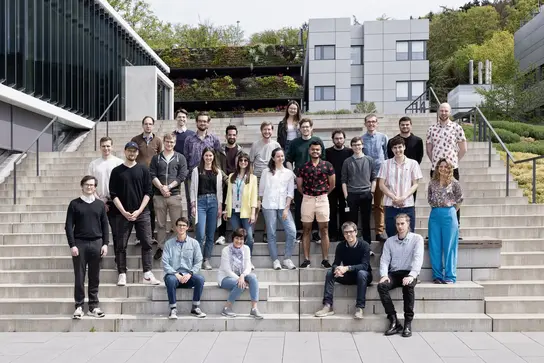
Selected Publications
Clarke, B., Holtkamp, E., Öztürk, H., Mück, M., Wahlberg, M., Meyer, K., Munzlinger, F., Brechtmann, F., Hölzlwimmer, F. R., Lindner, J., Chen, Z., Gagneur, J., & Stegle, O.
Marconato, L., Palla, G. , Yamauchi, K. A. , Virshup, I., Heidari, E., Treis, T., Toth, M., Shrestha, R. B., Vöhringer, H., Huber, W., Gerstung, M., Moore, J., Theis, F. J. , & Stegle, O.
Kleshchevnikov, V., Shmatko, A., Dann, E., Aivazidis, A., King, H. W., Li, T., Elmentaite, R., Lomakin, A., Kedlian, V., Gayoso, A., Jain, M. S., Park, J. S., Ramona, L., Tuck, E., Arutyunyan, A., Vento-Tormo, R., Gerstung, M., James, L., Stegle, O., Bayraktar, O. A.
Velten, B., Braunger, J.M., Argelaguet, R., Arnol, D., Wirbel, J., Bredikhin, D., Zeller, G., Stegle, O
Jerber, J., Seaton, D. D., Cuomo, A. S. E., Kumasaka, N., Haldane, J., Steer, J., Patel, M., Pearce, D., Andersson, M., Bonder, M. J., Mountjoy, E., Ghoussaini, M., Lancaster, M. A., Marioni, J. C., Merkle, F. T., Gaffney, D. J., & Stegle, O.
Bonder, M. J., Smail, C., Gloudemans, M. J., Frésard, L., Jakubosky, D., D’Antonio, M., Li, X., Ferraro, N. M., Carcamo-Orive, I., Mirauta, B., Seaton, D. D., Cai, N., Vakili, D., Horta, D., Zhao, C., Zastrow, D. B., Bonner, D. E., Wheeler, M. T., Kilpinen, H., … , Stegle, O.
Get in touch with us
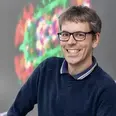
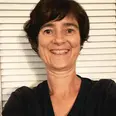
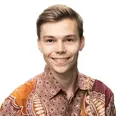