Tumor Immunology and Tumor Immunotherapy
- HI-TRON Mainz
- Immunology, Infection and Cancer

Prof. Dr. Niels Halama
Integration of broad aspects of immunological parameters for the successful development and optimization of therapeutic approaches in clinical translation.
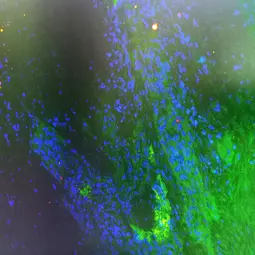
Our Research
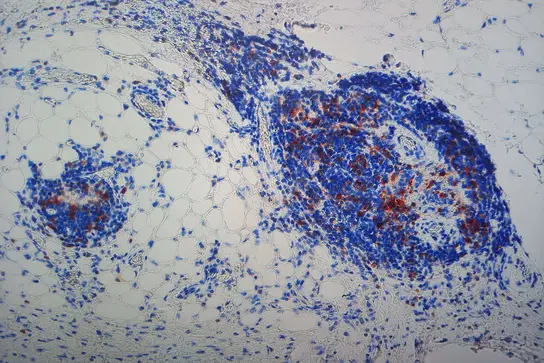
Cancer immunology has moved from scientific insight into practice changing standards in oncology. Robust scientific evidence has provided the entry to successful clinical translation. The department Tumor Immunology and Tumor Immunotherapy is integrating broad aspects of immunological parameters (i.e. tissue-specific regulation, microbiome, metabolic regulation, genomic factors etc.) for the successful development and optimization of therapeutic approaches in clinical translation. An important part is to better understand the regulation of immune responses within tissues of solid tumors. Dedicated biomarker research for the identification of relevant immune cell phenotypes is also a decisive factor like understanding the cytokine regulatory network in specific disease situations. Deeper insights into the situation in human patients are provided by our fully-human preclinical Explant Model System, which is able to recapitulate the microenvironment of individual patients. In addition, new computational model systems, databases and machine learning (“artificial intelligence”) allow to further elaborate our understanding of the regulation of immune responses. Especially our explant model systems open new possibilities in development of new therapies. Newly developed therapies have been successfully translated into the clinic and combinatorial immunomodulation based on adaptive clinical trial protocols with biomarker driven selection programs are now being implemented.
Cancer immunotherapy has revolutionized oncology. As a standard of care for some cancer entities, broad application in the treatment of patients with hematologic and solid tumors has become a reality. Identification of patients for a specific immunomodulatory approach, identification of successful combinatorial immunotherapy (or combination with chemotherapy, radiation or other intervention) are all important next steps to be developed also together in the Helmholtz Institute for Translational Oncology (HITRON). Utilizing new model systems and informative trial designs, the connection of basic research and translational efforts make this area highly diverse and challenging, but show the potential for a future of personalized therapy in oncology.
Projects
The longstanding expertise in connecting insights from pre-clinical model systems with early clinical trials revealed multiple possible interventions and early clinical trials. Two examples for this systematic approach and successful translation encompass the modulation of the chemokine axis CCL5 and its receptor CCR5 (Halama N et al, 2016, Cancer Cell) as well as the inhibition of the cytokine SDF1alpha. Systematic analyses of the microenvironment utilizing the explant model system (Halama N et al, 2016, Cancer Cell) indicated possible synergies for classical checkpoint blockade combined with inhibition of CCR5. The corresponding trial (PICASSO, ClinicalTrials.gov Identifier: NCT03274804) was performed with concomitant serial biopsies, allowing to investigate the effects of anti-PD-1 (Pembrolizumab) and CCR5 inhibition in massively pre-treated colorectal cancer patients. Although the findings from the PICASSO trial are published, the parallel trial investigating the triple immunotherapy combination treatment (LUMINESCENCE, ClinicalTrials.gov Identifier: NCT04721301, PI Niels Halama) is still ongoing with 50 patients recruited (25 colorectal cancer and 25 pancreatic cancer, all microsatellite-stable). Combination of double checkpoint inhibitors (anti-PD-1, anti-CTLA4) with CCR5 inhibition showed promising synergistic effects, enhancing the anti-tumoral effect of macrophage re-polarization with enhanced activation of the adaptive arm of the immune system. Systematic analyses of serial biopsies and the microbiome in patients on trial show promising results, especially as there are ~20% of patients with disease control, making this sample set unique in an otherwise immunotherapy-unresponsive entity. Clinical follow-up and concomitant research are still ongoing. Another component of the tumor microenvironment is formed by fibroblasts, building a barrier for T cell infiltration and modulating the immune responses. Inhibition of the SDF1alpha has been reported to affect the tumor microenvironment via modulation of fibroblasts. The new approach of inhibiting specific cytokines through a RNA molecule (“spiegelmer technology”) was applied within the OPERA trial (KEYNOTE-559, ClinicalTrials.gov Identifier: NCT03168139, PI Niels Halama) and results were published in the Journal of Immunotherapy of Cancer. Here, the mode-of-action could be clarified and showed an activation of the adaptive immune system in a subset of patients with a specific myeloid cell population presumably being the drivers for the enhanced anti-tumoral effects.
The possibilities of algorithmic approaches in the detailed analysis of tissue images are addressed in this project. Automated classification of tissue image elements can be performed in multiple ways. Systematic analysis and optimization of selected features, optimizing the algorithmic backbone (ResNet, AlexNet etc.) and training and validating the convolutional neural network (CNN).
The analysis of colorectal cancer primary tumor cohorts and prediction of prognosis was performed and published in (Kather JN et al, 2018, PLOS Medicine). This publication was the most cited publication of the year 2019 of PLOS Medicine. This approach was then further developed into an effort to automatically identify microsatellite-instable lymphocyte-rich colorectal cancers, published in Nature Medicine (Kather JN et al, 2019, Nature Medicine). The current work with machine learning algorithms seeks to implement a platform that allows to stratify explant models and bioprints (see section 1.2.4) automatically. This will allow to systematically perturbate the model systems and automatically annotate the changes into a prognostic subgroup through machine learning. This can then be applied for identification of possible targets for intervention or to estimate the effects induced by a specific drug or intervention on the tumor microenvironment.
The increasing number of heterogeneous datasets or limited-size datasets (as for subgroups of specific cancer entities or specific rare cancer entities) make systematic analyses challenging. Heterogeneous datasets and limited number of cases inhibit systematic approaches in a way that can annihilate efforts in precision medicine. This problem is addressed in two approaches. Topological algebra and computational topology is a framework that can better resemble the deeply interwoven relationships of biology represented in datasets (Moraleda et al, 2020, Semin Immunol, Valous et al, 2020 Semin Immunol). Therefore, a non-sql graph database was implemented in Neo4j and contains heterogeneous complex data. Now, in the next steps, systematic application of computational topology algorithms allows to classify and cluster datasets in novel ways. In addition to this, efforts have been initiated to leverage quantum computing methodology. In short, specific formulation of questions in a biquadratic layout allow for improved optimization results. Based on the quantum annealer technology, the systematic analysis of graph networks is being explored and a first prototype has been developed, allowing further optimization with geometric algebra to link the localization of identified central nodes to larger branches of the graph. This investigation of quantum methodology is a joint project together with collaboration partners from Fraunhofer IKS (Munich). The quantum processing advantage for limited datasets is further investigated for machine learning, where first results have been obtained (Matic A et al, ArXiv, 2022)[AM3] [HN4] . Currently, preparations for an international proposal are underway, on one hand investigating the optimal hardware and algorithmic approach and on the other hand investigating the utility for given clinical subsets, e.g. triple-negative breast cancer.
Classical immunotherapies focus on the modulation or activation of the adaptive arm of the immune system. Although excellent therapeutic successes have been achieved for some tumor diseases, others do not respond at all. In order to better leverage the potential of immunotherapy, we explore the role of the innate arm of the immune system. Since the microbiome composition can strongly impact therapy outcome, we focus on the interplay of microbiome and innate immune system within the tumor microenvironment. In this frame, we developed a pipeline utilizing Nanopore technology for 16S sequencing to systematically assess microbiome composition. Furthermore, we apply specific and highly sensitive PCR assays for measuring key factors impacting the immune system and therefore patient prognosis. These include qPCR assays to quantify Fusobacterium nucleatum and its products colibactin, Bacteroides fragilis toxin and FadA. Beyond the classical microbiome analysis, our research also focuses on the investigation of the mycobiome. Systematic analyses are currently being carried out to delineate fungal species on the basis of existing databases such as UNITE and WARCUP. First results of the above depicted pipeline with samples from the LUMINESCENCE trial (see section 1.2.1) show promising results with cross-entity patterns associated with either good or bad prognosis or treatment outcome. To better understand the microbiome composition within tissue, another technology has been further developed: assembloids or bioprints are artificially created tissues with a defined content of cell populations (i.e. cancer cells, T cells, macrophages, bacteria etc.). The matrix or scaffold consists of a collagen and cytokeratin containing substance, where the cells of interest are placed inside. This miniaturization allows systematic side-by-side comparison of different conditions or compositions. The effect of distinct cytokine mixtures, bacteria or bacterial communities on defined populations of tumor and immune cells is analyzed. A first use case of this approach has been published recently (Ahmed A et al, 2022, Frontiers Immunology).
Team
A 14-person team consisting of biologists, molecular biologists, physicists and physicians is working on the development and optimization of new therapeutic approaches to successfully combat cancer.
-
Prof. Dr. Niels Halama
-
Dr. Azaz Ahmed
-
Dr. Anna Berthel
-
Dr. Silke Grauling-Halama
-
Florian Heininger
-
Dr. Xu Holtkotte
-
Alicia Höflich
-
Karla Kerekovic
-
Alexandra Pöchmann
-
Dr. Heiko Weyd
-
Silke Will
-
Jana Wolf
-
Xin-Wen Zhang
Selected publications
Wankhede D, Yuan T, Kloor M, Halama N*, Brenner H, Hoffmeister M
Jahangir CA, Page DB, Broeckx G, … Halama N*et al.
Ahmed A, Köhler S, Klotz R, Giese N, Hackert, T, Springfeld C, Jäger D, Halama N*
Halama N*, Zoernig I, Berthel A, Kahlert C, Klupp F, Suarez-Carmona M, Suetterlin T, Brand K, Krauss J, Lasitschka F, Lerchl T, Luckner-Minden C, Ulrich A, Koch M, Weitz J, Schneider M, Buechler MW, Zitvogel L, Herrmann T, Benner A, Kunz C, Luecke S, Springfeld C, Grabe N, Falk CS & Jaeger D
Get in touch with us
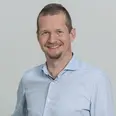
Prof. Dr. Niels Halama
AbteilungsleiterPostal address:
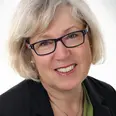
Marion Drechsel
Office ManagerPostal address: