Computational Patient Models

Dr. Kristina Giske
Research Group Leader
Our team is shaping digital patient twins tailored for radiotherapy. At the interface of advanced imaging, biophysical organ and tissue modeling, and artificial intelligence we explore subtle treatment responses using spatio-temporal-resolved in-silico patient replicas.
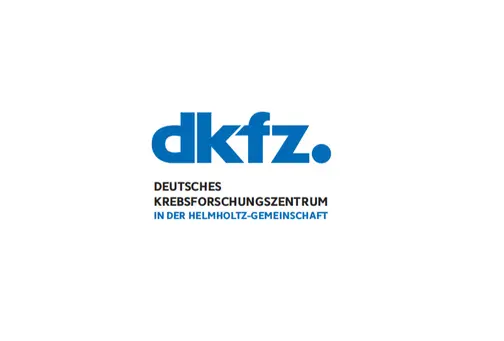
We are physicists, medical informaticians, data scientists, mathematicians, and radiooncologists working together for the benefit of the patient.
Our research is dedicated to in-silico radiotherapy simulation of cancer patients undergoing curative irradiation treatment. The first challenge for technically precise radiation therapy lies in adapting dose delivery to deformations in the patient’s anatomy induced by motion and physiological changes. The second challenge is to detect subtle changes in longitudinal imaging, which allow to test treatment outcome prediction models.
Our vision is to create a virtual patient model, serving as a proxy for treatment simulation by accumulating the physical dose and predicting the therapeutic effect of the planned treatment. This together empowers treating physicians to tailor the treatment to the specific needs of the patient before irradiation and during the treatemtn course. In-silico model development, advancements in imaging and computer technology, and our passion for unusual solutions are our tools in the fight against cancer.
Projects
MIRROR - Mobile Illustrator of Radiotherapy course for Review, data Orchestration, and Reflection
Radiotherapy is often complex and difficult for patients to understand, as it involves the integration of numerous pieces of information. Currently, neither patients nor their treating physicians-particularly primary care doctors-have straightforward access to all relevant data. Patients can usually view their treatment plans only on-site and lose access afterward.
In this NCT-funded project, the team is exploring the visualization concepts for a "digital twin"- a browser-based software tool that allows patients to access all essential information, such as imaging data and medical decisions, in a comprehensible format, regardless of location.
Team: Pedro Joao Rodrigues (DKFZ), Kristina Giske (DKFZ), Martin Niklas (DKFZ)
Collaborators: Fabian Weykamp (UKHD, KKE DKFZ)
CLARITY - CineMR-guided ML-driven breaAthing models for adaptive RadIoTherapY
Dose escalation in breathing anatomies of ultra-central lung cancer lesions is based on cutting-edge LINAC-integrated imaging technology using 2D cineMR time series combined with high-end adaptation strategies. Yet, the 4D motion trajectories still cannot be visualized and processed online with available technologies.
In this Helmholtz Imaging-funded project our team investigates biomechanical and ML-based 4D motion modelling approaches for their ability and reliability to predict critical deformation events of organ at risks from 2D cineMR.
Team: Richard Häcker (DKFZ), Kristina Giske (DKFZ), Oliver Jäkel (DKFZ), Daniel Lang (HMGU), Julia Schnabel (HMGU, TUM)
Collaborators: Sebastian Klüter (UKHD), Sebastian Regnery (UKHD), Juliane Hörner-Rieber (UKHD)
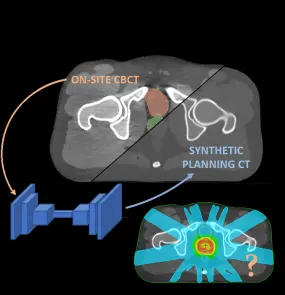
CBCTart - Data-Driven CBCT Image Quality Improvements for Online Adaptive Radiotherapy
CBCT imaging has become an integral component of photon radiotherapy devices. However, its image quality is restricted in its ability to faacilitate precise patient positioning. The primary objective of the project is to bridge the gap toward achieving image quality comparable to planning CTs, thereby enabling on-couch treatment plan adaptation based solely on CBCTs.
In this Varian-funded project the team investigates the impact of typical image quality shortcomings of CBCT on the degradation of plan quality. Additionally, generative deep learning models are employed to generate synthetic CTs from the lower-quality CBCT images.
Team: Goran Stanic(DKFZ), Kristina Giske (DKFZ), Niklas Wahl (DKFZ), Florian Ebert (DKFZ), Oliver Jäkel (DKFZ)
Collaborators: Fabian Weykamp (UKHD, KKE DKFZ), Bálint Kovács (MIC DKFZ)
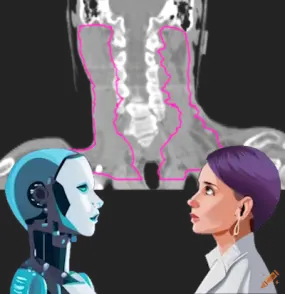
TVoracle - Towards Expert-Guideline Conformance for Machine-Learning-based Segmentations of Clinical Target Volumes
Patient-tailored contours of target volumes are crucial for radiation treatment planning, significantly influencing the outcome of cancer treatment. Clinical target volume delineation on planning CT scans poses challenges for human experts, proving to be extremely time-consuming and displaying substantial variation between observers. State-of-the-art machine learning algorithms achieve high accuracy on the automatically segmenting anatomical structures, but this success doesn't seamlessly extend to target volumes without additional constraints. The translation of expert guidelines into the machine learning realm has the potential to advance automated target volume delineation, facilitating guideline conformance and clinical use.
In this HIDSS4Health-funded project the team tackles the implications of supervised learning on data prone to inter-observer variabilities and patient-individual differences utilizing mathematical rules provided by human experts.
Team: Alexandra Walter (KIT, DKFZ), Jakob Kreft(DKFZ), Marc Buckmakowski (DKFZ), Kristina Giske (DKFZ), Oliver Jäkel (DKFZ), Martin Frank (KIT)
Collaborators: Philipp Hoegen-Saßmannshausen (UKHD), Sebastian Adeberg (UKHD)
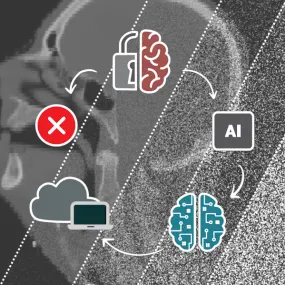
ALIEN - Generating non-existent Radiotherapy Patient Cousins for FAIR Research
Reseach progress in medicine relies on patient-sensitive data, particularly in radiation therapy, which involves the most diverse and frequent imaging requirements for each patient within its precise image-guided adaptive treatment options. Sharing such rich connected data in compliance with ethical and legal requirements necessitates thorough preparations in long-term projects. Technological short-term projects cannot share in-house data, preventing a direct comparison of competing algorithm designs. Creating a data cohort of non-existent but patient-representative radiotherapy-relevant scans could be made openly accessible for radiotherapy research.
In this AI Health Innovation Cluster-funded infrastructural project our team scrutinises cutting-edge AI image generator technologies for their capability to mimic medical scans with consistently realistic patient anatomies.
Team: Pedro Joao Rodrigues (DKFZ), Jule Katzenberger (DKFZ), Kristina Giske (DKFZ), Oliver Jäkel (DKFZ), Jens Fleckenstein (UMM)
Collaborators: Martin Niklas (DKFZ)
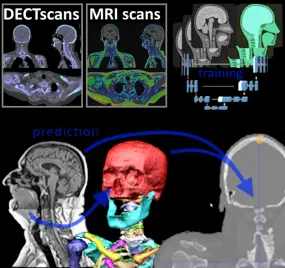
DeepSPYN - Deep Learning based PseudoCT Generation for MR-only Treatment Planning
Stopping power ratio (SPR) maps are essential for dose deposition calculations in ion beam cancer treatments and are usually estimated from single energy CT (SECT) in clinical practice. Dual-energy CT (DECT), which involves the acquisition of two energy spectra, captures both material-specific information and tissue characterization, showing promise in enhancing patient-tailored SPR map conversion.
In this BMBF*-funded project our team scrutinises the capability of deep learning models to convert MRI scans into pseudo-CTs or pseudo-SPR maps.
Team: Ama Katseena Yawson (DKFZ), Nora Wolf (DKFZ), Kristina Giske (DKFZ)
Collaborators: Thomas Welzel (UKHD), Katharina Seidensaal (UKHD), Stefan Dorsch (DKFZ), Katharina Maria Paul (DKFZ), Habiba Salem (UKHD), Julia Bauer (UKHD)
*ARTEMIS Project (#13GW0436B) in collaboration with UKHD & HIT (Jürgen Debus, Oliver Jäkel)
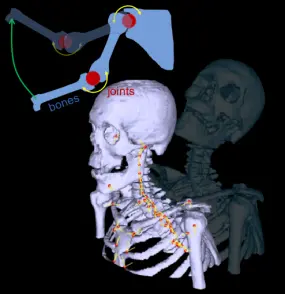
BionicDIR - Towards Biofidelic Deformable Image Registration of the Skeleton by Kinematically Articulated Multi-Body Physics
Accurate motion estimation between medical scans of different modalities (e.g. CT, MRI) is crucial for adaptive radiation therapy. The widely used intesity-based deformable image registration is limited by the absence of physical constraints during deformation. The KinematicDIR project studies the use of a biomechanical model for biofidelic image registration. For the head and neck area, it focusses on a kinematically articulated skeleton model as the driving motion model.
In this BMBF*-funded project our team investigates the best approach to incorporate the body model into the registration task and assesses the accuracy and robustness - but also limitations - of the model-based approach.
Team: Cornelius Bauer (DKFZ), Sina Thürwächter (DKFZ), Kristina Giske (DKFZ)
Collaborators: Thomas Welzel (UKHD), Katharina Seidensaal (UKHD), Katharina Maria Paul (UKHD)
*ARTEMIS Project (#13GW0436B) in collaboration with UKHD & HIT (Jürgen Debus, Oliver Jäkel)
based on PuppetMaster preceding projects by Hendrik Teske, Kathrin Bartelheimer, Peter Lysakowsky, Stephen Schaumann, Jan Meis
PRELUDE - Towards Learning 3D Thorax Anatomy and its Motion Extrapolation from 2D Cross-section Time Series
Precise irradiation of lung tumors can be highly challenging due to cyclic respiratory motion and deformations of neighbouring soft tissues in the mediastinum. Continuous online measurement of this complex motion can currently only be acquired via 2D time series, such as cine-MRI sequences. However, 2D anatomy and out-of-plane local deformations complicate fast mitigation techniques and limit dose monitoring along the intrafractional irradaition.
In this scholarship*-funded project our team scrutinizes machine learning strategies to detect suddenlyemerging organs at risk in the scan and extrapolate 4D anatomy based on time-resolved 2D snapshots.
*HRH Princess Chulabhorn's 60th Birthday Anniversary Scholarship
Team: Pasit Jarutatsanangkoon, Richard Häcker, Goran Stanic, Pedro Joao Rodrigues, Kristina Giske
People (Raster)
-
Dr. Kristina Giske
Research Group Leader
-
Joao Pedro Dias Rodrigues
Staff Scientist
-
Ama Katseena Yawson
doctoral student
-
Alexandra Walter
HIDSS4Health doctoral student
-
Goran Stanic
doctoral student
-
Richard Häcker
doctoral student
-
Marc Buckmakowski
master's student
-
Nora Wolf
master's student
-
Cassandra Krause
intern + master’s student
-
Jule Lea Katzenberger
bachelor’s student
Alumni
Reza Seyed Seif | Sina Thürwächter | Florian Ebert | Jakob Kreft | Dr. Pasit Jarutatsanangkoon | Mark Arndt ❘ Vahdaneh Kiani ❘ Mikulas Bankovic ❘ Nora Wolf ❘ Dr. Cornelius Bauer ❘ Tom Vichtl ❘ Katrin Weigand ❘ Eric Volkmann ❘ Stephen Schaumann ❘ Mohammad Amin Rashid ❘ Peter Lysakovski ❘ Dr. Kathrin Bartelheimer ❘ Rachid Zeghlache ❘ Charlotte Bordt ❘ Dr. Hendrik Teske ❘ Dr. Markus Stoll ❘ Dr. Paul Mercea ❘ Angelika Czekalla ❘ Julian Suleder ❘ Simon Kirchhof ❘ Luis Fernando Paredes Ocampo ❘ Johannes Merz ❘ Daniel Schaubach ❘ Thomas Wollmann ❘ Jan Meis ❘ Anna Storz ❘ Nico Schweiger ❘ Sarah Grimm ❘ Henry Müssemann ❘ Angelika Laha ❘ Dr. Eva Stoiber
Selected Publications
Walter A, Hoegen P, Stanic G, Rodrigues JP, Adeberg S, Jäkel O, Frank M, Giske K. 2024 Segmentation of seventy-one anatomical structures necessary for the evaluation of guideline-conform clinical target volumes in head and neck cancers. Cancers 2024, 16(2), 415. doi.org/10.3390/cancers16020415 Preprints 2023, 2023121562. doi.org/10.20944/preprints202312.1562.v1
Yawson AK, Walter A, Wolf N, Klüter S, Hoegen P, ADebus J, Frank M, Jaekel O, Giske K. 2024 Essential parameters needed for a U-Net-based segmentation of individual bones on planning CT images in the head & neck region using limited datasets for radiotherapy application. Phys Med Biol. in press https://doi.org/10.1088/1361-6560/ad1996/
Bauer CJ, Teske H, Walter A, Hoegen P, Adeberg S, Debus J, Jaekel O, Giske K. 2023 Biofidelic image registration for head and neck region utilizing an in-silico articulated skeleton as a transformation model. Phys Med Biol. 68(9):095006
Niebuhr NI, Johnen W, Echner G, Runz A, Bach M, Stoll M, Giske K, Greilich S, Pfaffenberger A. 2019 The ADAM-pelvis phantom - an anthropomorphic, deformable, and multimodal phantom for MRgRT. Phys Med Biol. 64(4):04NT05
Stoiber EM, Bougatf N, Teske H, Bierstedt C, Oetzel D, Debus J, Bendl R, Giske K. 2017 Analyzing human decisions in IGRT of head-and-neck cancer patients to teach image registration algorithms what experts know. Radiat Oncol. 12:104
Teske H, Bartelheimer K, Meis J, Bendl R, Stoiber EM, Giske K. 2017 Construction of a biomechanical head and neck motion model as a guide to evaluation of deformable image registration. Phys Med Biol. 62(12):N271-N284
Teske H, Bartelheimer K, Bendl R, Stoiber EM, Giske K. 2017 Handling images of patient postures in arms up and arms down position using a biomechanical skeleton model. Current Directions in Biomedical Engineering 3(2):469-472
Bartelheimer K, Teske H, Bendl R, Giske K. 2017 Tissue-specific transformation model for CT-images. Current Directions in Biomedical Engineering 3(2):525-528
Stoll M, Stoiber EM, Grimm S, Debus J, Bendl R, Giske K. 2016 Comparison of Safety Margin Generation Concepts in Image Guided Radiotherapy to Account for Daily Head and Neck Pose Variations. PLoS One 11(12):e0168916
For a full list of articles, theses etc. from the division, see here.
Get in touch with us
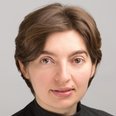