Digital prevention, diagnostics and therapy guidance
- Cancer Risk Factors and Prevention

Priv. Doz. Dr. Titus Brinker
Abteilungsleitung
The main goal of the group is the development of robust and interpretable digital biomarkers to improve prevention, non-invasive early detection, diagnostic, and therapeutic approaches.
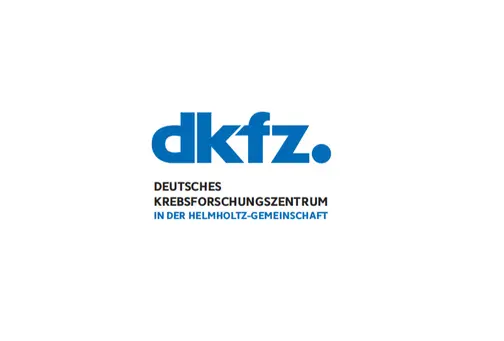
Our Research
A 20-member, almost fully externally funded team from the fields of medicine, molecular biology and informatics/data science focuses on identifying relevant patterns in patient data and increasing the explainability and robustness of deep learning-based classifications. We see software systems as part of clinical teams for more efficient patient care and at the same time as a tool for effective prevention and early detection. In the past (since 2020), we have achieved much-noticed scientific success in these areas; our more than 80 internationally peer-reviewed research papers have been cited more than 5,000 times and numerous project results have been picked up by international media. Software products or apps from our division have been downloaded more than a million times.
Seven recently approved grants include the MiRisk consortium (1) which develops a free app to individually determine and minimize the risk for breast cancer. Within the BAP-1-consortium (2), we share & extend our expertise in building histology image pipelines to stratify patients for drug development. The Hector grant (3) enables us to integrate spatial transcriptomics for deep-learning-based heterogeneity scores to predict melanoma metastasis. The sKIn project (4) takes the remaining technical and formal steps to build our dermatologist-like skin cancer AI into dermatoscopes together with a company, bringing them into the hands of caregivers. MELCAYA (5) identifies new risk factors for melanoma in CAYAs. A deep learning strategy for high-throughput proteomics (6) allows higher resolution and faster processing of liquid biopsies. A signed collaboration with industry will lead to more individualized sunscreen recommendations based on epigenetic tests read from smartphone photographs via AI. Improved digital analysis of sarcomas (7), the interaction of language models and care, explainable AI algorithms for cancer screening and the optimization of the Sunface & Smokerface App also depict future plans of the division.
On the following pages you can find out more about the people in our team, their main areas of work and the projects our division is currently working on.
Projects
.
Better Understanding of Risk Factors and Progression of Melanoma in Children, Adolescents and Young Adults to Implement Health Care Strategies (MELCAYA; European Commission)
Melanoma in childhood and adolescence is under-studied, lacking adequate preventive, diagnostic, and therapeutic strategies. The incidence of melanoma is reported to be about 1.3-1.6 per million in children under 15 years of age and 15 per million in 15-19 y.o., with increasing incidence in adolescents by 4.1% annually since 1997. Additionally, melanoma is among the most frequent solid tumors diagnosed in young adults, having in Europe an incidence of 6.6 per 100.000 and a mortality of 4 per million. Little is known about the interaction between genetic and environmental factors associated with melanoma risk in this context or in the progression from benign nevus to melanoma. As benign melanocytic lesions develop in nearly every child and are present in over 1% at birth, the diagnostic accuracy of melanoma is very low in this age group. A large number of benign lesions are unnecessarily excised (593.8 benign nevi per melanoma under 20 y.o.). Furthermore, lower sensitivity than in adults results in late diagnosis with poorer outcomes, when children, adolescents and young adults (CAYA) patients, particularly the children, could show a 90% 10-year survival rate from stage I/II disease. Thus, melanomas in CAYAs represent a medical challenge. Increasingly, machine learning algorithms are starting to play an important role in melanoma diagnosis. However, these algorithms are based on adult data with a selection bias that could be detrimental and potentially dangerous with respect to both melanoma underdiagnosis and overdiagnosis in CAYA.
Within a consortium of international experts in melanoma from diverse areas, international reference hospitals for pediatric and adolescent patients, AI data scientists, basic researchers, innovative technologic SMEs and patient associations, the Brinker group at the DKFZ is the PI for the development of strategies for digital prevention and early detection for melanoma in CAYAs.
The main aim of the overall project is to obtain insights into the mechanisms of melanoma development and progression in CAYA to develop novel strategies for prevention and innovative technologies for diagnosis and prognosis.
Characterization of the Intratumoral Heterogeneity of Melanoma by Spatially Resolved Molecular and AI-based Analyses (Hector Foundation, Germany)
The project is a collaboration with the Clinical Cooperation Unit Dermato-Oncology of DKFZ (Jochen Utikal). It focuses on understanding spatial intra-tumoral heterogeneity in malignant melanoma and its impact on patient prognosis. By studying both transcriptional and morphological heterogeneity, we aim to investigate the relationship between the different levels of heterogeneity, their correlation with prognosis, and potentially train an algorithm to identify relevant segments for potential use as digital biomarkers.
At the gene expression level, Xenium in-situ analysis will be employed to investigate transcriptional heterogeneity. Selected genes from mesenchymal and melanocytic signatures will be examined. Histologically, heterogeneous segments will be characterized using pathological annotation and AI-based clustering to further investigate correlations between molecular and histological properties. A Deep-Learning-based segmentation algorithm will be trained on fusion segments resulting from overlaps between the two categories to annotate spatial heterogeneity on new melanoma sections. If a correlation between the extent of heterogeneity and prognosis is found, the algorithm can be used to train a risk classifier as a digital prognostic biomarker to predict recurrence or mortality risk.
Heterogeneity, Evolution and Resistance of Fusion-driven Sarcomas in Adolescents and Young Adults (BMBF)
Tumors evolve through genetic and non-genetic changes, leading to intra-tumoral heterogeneity (ITH), a key factor in relapse and therapy resistance. Our focus is on bone and soft-tissue sarcomas caused by chimeric fusion genes. These serve as model diseases to investigate ITH in cancers with defined genetic drivers, translating findings into innovative treatment strategies against diverse and evolving tumors. Challenges include limited effective therapies, stagnant outcomes, and early onset, emphasizing the urgency to improve sarcoma patient outcomes. Fusion-driven sarcomas (FDS) offer a unique opportunity due to their entity-defining fusion genes, aiding the study of subclones and plasticity. FDS also exhibit low genetic complexity, facilitating the identification of ITH-promoting factors and responses to therapy. Leveraging the expertise of HEROES-AYA, an international precision oncology network, we aim to study FDS at diagnosis and during therapy resistance through cutting-edge analyses at single-cell and spatial levels. In vitro and in vivo validations of vulnerabilities will inform the development of clinical trials spanning age groups, ushering in a new era of precision oncology in Germany. The group substantially contributes to the data science aspects of this project.
Development of Digital Biomarkers for BAP1 Mutated Cancers (German Cancer Aid)
The project centers around BAP1, a tumor suppressor gene often mutated in aggressive cancers. Specifically, BAP1 mutations lead to increased tumor aggressiveness and metastasis. The goal of this project is to understand and target a vulnerability of BAP1 mutations for potential therapies in BAP1-mutant cancers. To achieve this, a collaborative consortium has been formed with subprojects focusing on providing necessary materials, testing specific drugs in vitro, in vivo and in patient-derived organoids, as well as conducting biomarker analyses on large human tissue cohorts. The overall aim is to validate the proposed mechanism and explore potential treatments, with an emphasis on initiating clinical trials based on the findings. The project integrates diverse approaches, including in vitro, in vivo, ex vivo, and clinical sample analyses, to comprehensively investigate BAP1-mutant cancers and develop personalized medicine strategies. Within this consortium, our group is responsible for digital biomarker development for improved patient stratification.
A Deep Learning Strategy for High-throughput Proteomics of Blood Plasma and Single Cells (Heidelberg Explorer Call)
This project is a cooperation with the proteomics laboratory of Prof. Krijgsveld. Proteins are the main drivers of cell function and disease, and therefore proteomics is a highly suitable technique to characterize determinants of cell identity and to identify biomarkers. Current proteomic technology has the breadth to profile thousands of proteins and the sensitivity to access single cells, however it lacks the throughput for meaningful analysis of large sample cohorts both in basic research and the clinic. Therefore, we here develop novel artificial intelligence (AI)-based approaches for the analysis of mass spectrometric (MS) data, to assign plasma proteomic data to clinical status, and to correlate single-cell data to cell identity. In addition, we will perform proteomic experiments to show that this deep learning-driven approach enhances throughput of plasma and single-cell proteome analysis. Collectively, this project will introduce a disruptive approach in proteomics to enable patient stratification and biomarker discovery, and to advance the young field of single-cell proteomics.
Team
20 Employees
-
Priv. Doz. Dr. Titus Brinker
Abteilungsleitung
-
Sarah Haggenmüller
Wissenschaftliche Projektleitung - sKIn
-
Max von Knobloch
Technische Projektleitung - sKIn
-
Franziska Schramm
Qualitäts- und Risikomanagerin
-
Tirtha Chanda
Software Scientist
-
Hendrik Alexander Mehrtens
Software Scientist
-
Ama Katseena Yawson
Software Scientist
-
Dr. Kevin Allen
Usability Engineer
-
Katja Hauser
Wissenschaftliche Mitarbeiterin
-
Julia Abels
Wissenschaftliche Mitarbeiterin
-
Dr. Sara Laiouar-Pedari
Wissenschaftliche Mitarbeiterin
-
Lukas Heinlein
Doktorand
-
Martin Joachim Hetz
Doktorand
-
Dr. Judith Hermanns
Data Scientist
-
Jana Winterstein
Doktorandin
-
Christoph Wies
Doktorand
-
Arlene Kühn
Wissenschaftliche Mitarbeiterin
-
Gesa Mittmann
Doktorandin
-
Carina Nogueira Garcia
Clinician Scientist Fellow
-
David Krauß-Roskamm
Wissenschaftliche Hilfskraft
Selected Publications
Chanda, T., Hauser, K., Hobelsberger, S., Bucher, T. C., Garcia, C. N., Wies, C., ... & Brinker, T. J.
Hetz, M. J., Bucher, T. C., & Brinker, T. J.
Brinker, T. J., Faria, B. L., de Faria, O. M., Klode, J., Schadendorf, D., Utikal, J. S., ... & Bernardes-Souza, B.
Get in touch with us
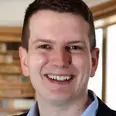